Editor's Note: This article is an update of an article originally written in 2010 by Dr. Vineet Jain, explaining the inner workings of one of the most critical (and difficult to develop) components of a catastrophe model—its damage functions.
While detailed hazard information is usually plentiful and often publicly available, relatively little data is available to construct damage functions.
Introduction
In catastrophe models, damage functions translate the intensity of a hazard affecting a structure (ground shaking, wind speed, water depth, etc.) to a damage ratio, which is the ratio of the repair cost of a building to its replacement value (cost to rebuild the entire structure). While detailed hazard information is usually plentiful and often publicly available, relatively little data is available to construct damage functions. It is thus not surprising that this component of the catastrophe model is considered one of the most misunderstood.
This article attempts to dispel some common myths about damage functions, such as:
- Damage functions are completely independent of other model components
- Damage functions for a given construction type (and peril) are very similar across countries and regions
- Engineering analysis is sufficient to develop damage functions
- Damage functions are deterministic in nature; once the wind speed is known, for example, it is possible to calculate damage severity with certainty
- All structures within a modeled hazard footprint suffer damage at damaging wind speeds or ground shaking
- Changes in building codes have an immediate effect on vulnerability
What Is a Damage Function?
In catastrophe modeling, damage functions—or, more precisely, mean damage functions—relate the hazard intensity to the mean damage ratio (MDR), which is the expected value of the ratio of the repair cost of the asset to its replacement value.1 In principle, damage ratios can exceed 1.0 because it can cost more to repair a building than to replace it.
Damage functions are also referred to as vulnerability functions or vulnerability curves, and actuaries refer to them as loss functions because they yield insurance losses. Fragility functions can be used as an alternative but not as a substitute; they are not the same as damage functions. Fragility functions (also called fragility curves) express the probability that an asset will reach or exceed a clearly defined limit state or damage threshold (from visible cracking and loss of roof covers to catastrophic collapse, for example) as a function of some measure of environmental excitation (typically a measure of acceleration/deformation or force in an earthquake, hurricane, or any other extreme loading condition). In contrast, damage functions help obtain losses in terms of repair costs, life safety impacts, and loss of functionality (dollars, deaths, and downtime), or in terms of environmental degradation, quality of life, historical value, and other measures.
Figure 1 shows representative mean wind and flood damage functions for a wood frame structure.
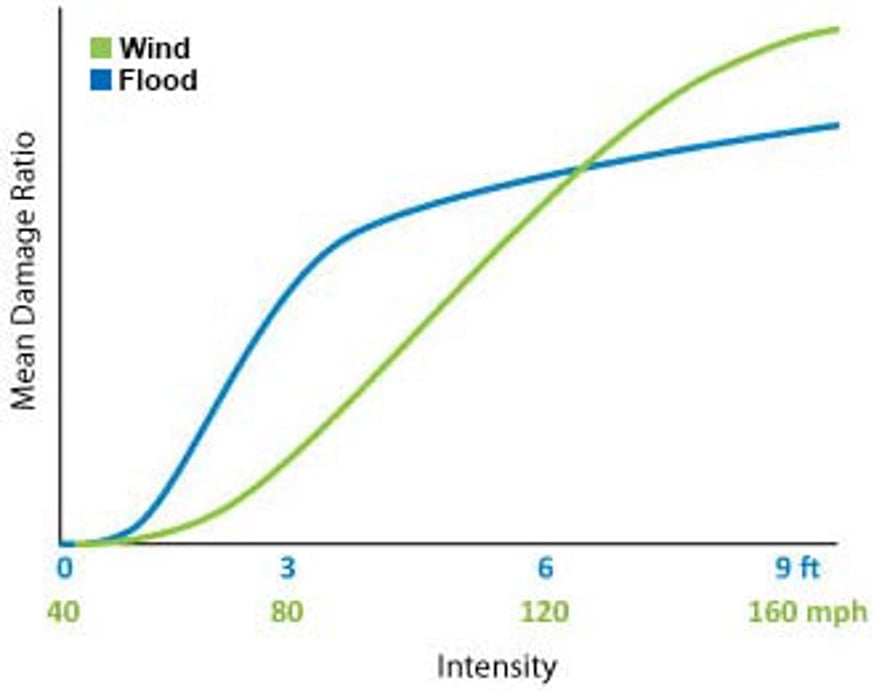
Figure 1. Schematic of wood frame mean damage functions for wind and flood.
As evidenced by the shape of the damage functions in the figure, the response of a structure varies significantly with the type of hazard being modeled. Flood damage to a wood frame home typically increases rapidly after water depth exceeds about 1 foot, but increases more slowly after 3 to 5 feet of water. In contrast, damage from wind is slow to accumulate until wind speeds reach about 90 to 100 mph; thereafter, damage increases rapidly. A structure’s overall vulnerability is also peril-dependent. While the heavy roof tiles seen on many homes in Japan can effectively resist the uplift forces imposed by typhoon winds, they magnify the loads (and therefore damage) imposed by an earthquake.
Beyond Engineering—Estimation of Future Payouts
While engineering is at the core of a catastrophe model’s damage function development framework, the ultimate objective of a catastrophe model is not the estimation of physical damage states or idealized cost estimates; rather, it is the estimation of future monetary losses that an insurer will actually pay out in the aftermath of a catastrophe. To achieve this, factors other than a structure’s engineering response must be accounted for, such as region and age, local building codes and their enforcement, claims adjustment and insurance practices, socioeconomic effects, and societal preparedness and response, to mention a few, as will be described in the following sections.
Regional Building Codes: Strength, Adoption, and Enforcement
The vulnerability of structures is highly location-dependent not only due to differences in their strength (hazard maps that govern the design of buildings), but also due to differences in the local adoption and enforcement practices of building codes, which varies significantly around the world. While state and local building code departments can relatively quickly adopt the latest building codes, there is typically a lag in actual implementation as a result of limitations in financial, training, and personnel resources in addition to the length and stringency of the procedures required by the legislature.
In extreme cases, building codes may not be required to be adopted at all, and indeed several jurisdictions in Mississippi currently do not require buildings to be designed under any building code. Code enforcement practices may also vary spatially and temporally. For example, within U.S. hurricane states, code enforcement is more stringent in the coastal counties because they have a higher risk, although a department’s financial resources, which are used for code official/inspector salaries and training, also come into play. These differences can have a significant effect on losses. (To learn more about the impact of evolving building codes on damage functions, please read “U.S. Model Update Enhances View of Wood Frame Vulnerability”)
Claims Adjustment and Insurance Practices
Even when buildings are similar across regions, there may be differences in how claims are settled. Insurance companies in some regions, for example, can be less “customer friendly” and therefore less ready to settle claims compared to insurance companies in other regions, which issue reassuring statements about claims settlements even before the winds have died down. Damage functions must account for settlement practices because they impact the final claims paid.
Whether policies cover replacement cost (typical for homes and businesses) or actual cash value (typical for automobiles) is another factor that affects how damage functions are formulated.
Socioeconomic Effects
Risk perception and risk tolerance can vary significantly across regions. A small crack caused by an earthquake to the wall of a home in California may elicit a very different response from the homeowner than will the same crack in Mexico, and thus lead to different monetary payouts. Socioeconomic conditions can also be an important predictor of the response of a structure under loads induced by a catastrophe. A wood frame building in a low-income neighborhood will not, in general, be as well maintained—or resilient—as a similar building in a higher-income neighborhood.
Preparedness and Response
How a business owner prepares for a catastrophe can significantly impact losses. In particular, business interruption losses can be significantly mitigated if management is well prepared and has worked with suppliers and customers to reduce business downtime. Preparedness at the local and regional levels, such as having plans in place to restore lifelines (e.g., utilities) quickly, can further limit losses.
Representation of Hazard
How damage functions are formulated in a model also depends on how the hazard is represented. For example, damage functions can be developed separately for each sub-peril within a larger peril (e.g., direct wind, wind-borne debris, wind-driven rain, and precipitation-induced flood for hurricane), or they can be developed to reflect combined risk based on one or two hazard variables. If the sub-perils are well-correlated and their modeling is computationally intensive, it may not be worthwhile to develop separate functions for each, especially if the sub-peril is typically covered automatically in standard policies for the particular region.
Hazard intensity can be represented in several ways: for example, for hurricane winds, it can be maximum sustained 1-minute wind speed, peak 3-second gust wind speed, or maximum mean wind speed measured either continuously over the full duration of a hurricane or at discrete time intervals. How the model’s damage functions are constructed will depend upon what measure of intensity and what resolution is used to calculate losses. Therefore, one must be careful when comparing the damage functions of different models.
Finally, while all components of a robust catastrophe model should be independently developed and validated to the extent possible, it remains a model, which means that simplifying assumptions must be made. In the end, the goal of the model is to produce reasonable and reliable loss estimates. To achieve that, it is calibrated to real life experience. During that calibration process, uncertainties can inadvertently spill over from one component to another and an implicit dependence between hazard and vulnerability is introduced. It does not, however, lessen either the value or the integrity of the model.
Damage Functions Capture Uncertainty
There is a tendency for users of catastrophe models to ask for—and expect—“the number”: given that wind speeds at location X were Y mph, what are my losses at that location? By now, however, the reader will recognize that damage—and therefore damage functions—are influenced by both complex human actions and the almost infinite complexities of the natural phenomenon being modeled.
These complexities conspire to make “the number” an unobtainable goal. Even the most sophisticated wind-measuring instruments are associated with errors on the order of 5-10%. This can translate to errors in damage estimation on the order of 50-100%. Predicting human behavior in response to a catastrophe is even more uncertain. Capturing these uncertainties in the model’s damage functions is critical—and challenging.
When judging model performance, comparisons are often made, unfortunately, between the modeled mean losses and the observed losses for those locations—but only for those locations that have resulted in actual claims. This comparison has built-in survivorship bias and is not statistically valid. For locations with claims, modeled mean losses are generally smaller. However, it is important to note there may be many locations with zero claims but a non-zero modeled mean loss. A more meaningful comparison would be to consider both the aforementioned cases and evaluating for any systematic bias between actual and modeled mean loss—including those locations without any actual loss. Alternatively, stochastic realizations of location-level modeled losses using the damage probability distribution in the model can be generated and statistical analyses be performed comparing these realizations to actual claims experience. It should also be noted that because of all the sources of uncertainty involved, it is much harder to predict precise losses at a given location for a given event. Due to the law of large numbers, this uncertainty decreases quickly as the number of locations in a portfolio increases.
Indeed, the main purpose of catastrophe models is not to predict losses at a location for a given event, but rather to provide reliable estimates of potential losses to properties or portfolios of properties from future catastrophe experience, and to effectively differentiate the vulnerability of properties in different regions.
Damage Function Development
Given the underlying complexities that underpin a catastrophe model’s damage functions, a natural question is: How are they developed? Multiple approaches have been used at AIR. If unlimited empirical claims data for all possible construction types and locales were available, then statistical approaches would suffice. On the other hand, if there were no data at all, then purely engineering-based approaches would be the only recourse. Reality is always somewhere between these two extremes, and which approach carries more weight depends on the availability of data for each region and peril. Claims data in regions with robust and mature insurance markets will clearly be more abundant than in regions where insurance markets are still emerging; similarly, for reasons of their relative frequency, claims data for hurricanes is far more abundant than claims data for earthquakes.
In general, damage functions are first estimated based on engineering analysis, research publications, and damage survey and loss data. As more loss data becomes available, the damage functions are re-evaluated and updated as necessary. This process is continual, as the vulnerability of the building stock keeps changing over time.
Closing Thoughts
The role of damage functions in a catastrophe model is to connect the latest science and engineering to real-world outcomes. While engineering is at the heart of damage function development, engineering alone cannot capture the myriad factors that determine monetary losses. Some of these factors are known, but others are not—and may indeed be unknowable. Furthermore, the target is always moving, as vulnerability is constantly changing regionally and over time.
An informed understanding of the complexities that go into the development of a catastrophe model’s damage functions and what they are meant to capture can only lead to a more informed understanding—and better use—of model output.
References
1 Repair costs are commonly normalized by replacement value, or the cost of a similar new property having the nearest equivalent utility as the property being appraised, as of a specific date (American Society of Appraisers 2013). Replacement value excludes land value and usually refers to part or all of the fixed components of a building or other asset (structural, architectural, mechanical, electrical, and plumbing components) or to its contents.