The political changes of 2016 surprised many people, especially some who put their trust in surveys. Many of the U.S. elections polls were wrong, just as the Brexit polls were wrong earlier in the year. All of this may somewhat undermine faith in people who make predictions for a living, including pollsters and actuaries. So the question is, are actuarial predictions subject to the same risk as election predictions?
To answer that question, we begin with a brief review1 of how some pollsters make their predictions.
- Random sample: To start the process, a very small sample of the population is surveyed, generally comprising people randomly chosen from each geographic area (as defined by area code). Pollsters use different methods to collect a random sample, including phone and email.
- Survey design: The wording and order of questions are also carefully considered. Pollsters often try to avoid leading questions where other-than-neutral phrasing could affect someone’s response. They also try to ensure that respondents aren’t primed to answer a certain way. For instance, a question about a candidate’s misdeeds should not immediately precede a question about whom you plan to vote for.
- Data collection and analysis (oversampling): Due to the huge variances in preference across demographics associated with small sample sizes, even a minor change in the demographic mix could cause a notable difference in the overall poll. With this in mind, pollsters tend to oversample (“adjust”) the data to make the results stable. Further, pollsters don’t provide data on the demographic mix of their polls; therefore, it’s difficult to characterize the bias for their samples.
Problems with recent polls
Despite these best practices, errors did plague some recent polls.
One error was a result of the sampling assumptions. Polling is a social science, not a natural science; and it’s harder to predict human behavior than natural phenomenon. Simply put, the past isn’t always predictive of the future. For example, in the 2016 election, many more people in rural parts of the country voted than were assumed in some of the samples used to make the election predictions.
Obtaining a representative sample was also more challenging. Traditionally, pollsters have found representative samples by calling published home phone numbers. But as more people rely on unpublished cell phones, pollsters have faced new challenges2 defining samples. Online polls have helped and present a cheap and fast alternative, but that also has challenges with estimates indicating 13 percent3 of the population doesn’t use the Internet.
Data problems also affected the accuracy of 2016 election predictions. Some respondents didn’t disclose that they planned to vote for a particular candidate (perhaps due to concern of being judged for their choice), which resulted in underestimating how many electoral votes that candidate would receive. Additionally, some people simply couldn’t be reached (for example, aforementioned rural populations may be more difficult to be contacted by phone), which biased the sample.
Actuarial science vs. polling
Is actuarial science social like polling, or is it a natural science? It’s arguably like both: insurers’ results are ultimately the result of people’s behaviors, but behaviors (and results) are also influenced by natural phenomena such as weather. To make loss cost predictions, actuaries at ISO analyze data submitted to ISO by insurance companies each quarter. Companies report data on premiums and losses, using detailed instructions in ISO statistical plans, which in turn are based on the data elements in classification plans that insurers use to rate policies. This data reported is generally large and representative enough such that only minimal recalibration is necessary. ISO actuaries then cleanse the data, apply assumptions to bring the past data to projected future levels, express the data as an estimated cost per unit exposure, and communicate any material differences from cost levels currently in effect to insurers through loss cost review circulars.
It’s debatable whether actuarial predictions are subject to the same risks as election predictions. Because loss cost reviews are generally conducted annually or more frequently and minimal sampling is applied, they can respond more quickly to changes in underlying demographics than, say, presidential election polls. That said, there are technological changes (for example, moving to vehicle autonomy) that we wouldn’t necessarily be able to completely reflect by purely looking at the recent past of data reported. Our findings show for the most part (using a sample of states for personal auto) that looking at the past was predictive of the future a large percentage of the time (see Exhibit 1).
Poor-quality data is also a challenge in modern actuarial work. Things can go wrong in at least two areas of insurance ratemaking. First, prospective insureds may not always accurately report basic facts about themselves during policy quotes. For example, auto applicants may only guess their annual mileage.
These issues tend to manifest at a micro level but in a macro sense don’t necessarily change significantly over time (with some exceptions, for example, economic crises that can create incentives for soft fraud).
Errors and inconsistencies can also occur when companies record data. As data elements considered in insurance rating change over time (for example, new classification plans), different levels of segmentation are required. Insurers can’t necessarily retrofit old data to the latest or best approach—requiring assumptions to be made.
Actuarial standards of practice exist for areas such as risk classification and data quality that outline best practices and required considerations. ISO makes adjustments to the data received from insurers according to those standards when performing loss cost reviews and advises insurers on the quality of their data submitted4. The risk of prediction error is mitigated accordingly.
So, are actuaries any better at predicting the future than political pollsters?
We’d like to think so. But, as with elections, the only way to address the challenges of data harvesting in actuarial science is to work as hard as possible to gather the most objective data possible and analyze it in the most complete way. That means looking at lots of data, making sure the data is high-quality, using multiple techniques and measures to analyze the data, and finally, looking at the results to monitor whether the working assumptions still apply.
If you have any questions, please feel free to contact me at YZhou@verisk.com or 201-469-2734.
Exhibit 15
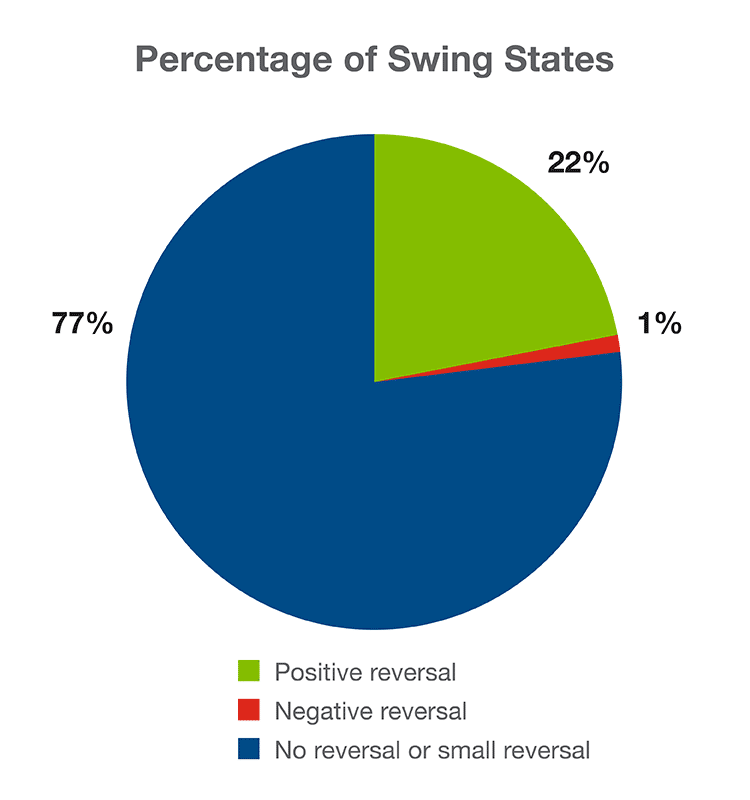
Exhibit 1 evaluates consistency of ISO personal auto loss cost indications from year to year. In this chart, we’re comparing each state’s all-coverages-combined indication of the current year with the previous year’s indication and grouping the differences into intervals. We can see that more than three-quarters of the states are very consistent in terms of the loss cost indications in the connecting years, with less than 5 percent moving in the opposite direction. For the 22 percent of loss cost reviews with indications that did move more than 5 percent in the opposite direction, this may reflect discrete exogenous factors as opposed to forecasting difficulties. For Oregon, a new law enacted in January 2016 concerning personal injury protection insurance and uninsured/underinsured motorist insurance reportedly results in higher claim costs, which has the potential to boost the loss cost indication that ISO predicts.
Are electoral and insurance predictions correlated?
As a small-scale test of whether electoral and insurance predictions are correlated, we identified all states that had ISO personal auto loss cost changes of 5 percent or more in the most recent year. We then compared the percentage of the population in those states that voted for the Republican U.S. presidential candidate in 2016 versus 2012. Despite the country as a whole casting more Republican votes in 2016 versus 2012, the majority of those particular states with auto loss costs changes of 5 percent or more had at least 2.5 percent fewer people voting for the Republican candidate in 2016 than in 2012.
This is probably a coincidental finding, and we raise it not for purposes of suggesting any sort of linkage between political preference and insurance losses, but rather to give deeper consideration to the role of nontraditional data in actuarial prediction as a supplement to historical insurance data. Just as pollsters use “polls plus” predictions that combine economic indicators with polling data, there has reportedly been at least one insurance company patenting potential uses of election data in ratemaking—although we may not see this anytime soon.6
At ISO, we’ve used risk-related non-insurance data such as weather and traffic to help develop ISO Risk Analyzer®, a suite of predictive models that help carriers classify, segment, and price their insurance risks. These tools examine hundreds of indicators and predict expected losses at the policy level by major coverage or peril. It’s currently available for the following lines: homeowners, personal auto, commercial auto, and businessowners.
State | Most recent year loss cost level change7, 8 | Difference in percent votes for non-incumbent party candidate in 2012 and 2016 |
---|---|---|
Arizona | +8.0% | -4.98% |
Colorado | +6.1% | -2.88% |
District of Columbia | +8.4% | -3.21% |
Florida | +18.6% | -0.11% |
Georgia | +15.1% | -2.53% |
Kansas | +7.6% | -3.06% |
Louisiana | +8.3% | 0.31% |
Maine | +7.3% | 3.89% |
Maryland | +7.2% | -1.99% |
New Mexico | +6.1% | -2.80% |
North Dakota | +5.7% | 4.64% |
Oregon | +5.7% | -3.06% |
South Carolina | +5.9% | 0.38% |
South Dakota | +7.3% | 3.64% |
Tennessee | +9.1% | 1.24% |
Utah | +10.1% | -27.25% |
Virginia | +5.9% | -2.85% |
Washington | +5.7% | -3.22% |
Wyoming | +6.1% | -1.24% |
Source:
1Dan Cassino. "How Today's Political Polling Works" Harvard Business Review. August 10, 2016
2Vann R. Newkirk II. "What Went Wrong With the 2016 Polls" The Atlantic. November 9, 2016
3Monica Anderson and Andrew Perrin. "13% of Americans Don't Use the Internet. Who Are They?" Pew Research Center. September 7, 2016
4Dennis Huang and Richard Morales. "High Quality Analytics from High Quality Data: the ISO Data Quality Review Process" Retrieved December 22, 2016
5ISO preliminary indication circulars
6Federal Elections 2012 Election Results for the U.S. President, the U.S. Senate, and the U.S. House of Representatives
7Leip, David;“2016 Presidential Election Results”;Dave Leip’s Atlas of U.S. Presidential Elections. Retrieved;December 19,;2016.
8Cicero. "What's After Safeco's Patent Linking Voter Behavior to Insurance Claims?" Prweb. November 10, 2012.