Editor's Note: This article is the second in a series of four articles that Verisk will publish every few months about our Next Generation Modelling (NGM) initiative. Part I discussed our new loss accumulation methodologies with spatial correlations. The next two in the series will discuss the following topics: the propagation of uncertainty to commercial lines—loss accumulation and complex (re)insurance structures; and the next generation of direct treaty and facultative loss module. In this article, we discuss the implementation of our new loss accumulation methodologies for residential and small business lines and how we model secondary uncertainty loss distributions, coverage correlations, and single risk terms. We conclude by discussing financial modelling for secondary perils, as applicable to residential and small business lines, using the next generation financial module.
We have improved the estimation and validation of the secondary uncertainty distributions for this modelling task using industry claims for all insurable secondary perils
Insurance for the residential and small business segment is characterized by single risk, single tiered policies. Verisk’s Next Generation Modelling Framework offers enhanced modelling of insurance coverage, secondary uncertainty distributions, and loss propagation to the geographical and portfolio levels. Because modelling insurance coverage of secondary perils has increased in importance for the industry, we have improved the estimation and validation of the secondary uncertainty distributions for this modelling task using industry claims for all insurable secondary perils. To properly account for policy terms and conditions, we have improved the actuarial accuracy of uncertainty propagation during the loss calculation process. In Part I of our NGM series, we discussed how portfolio rollup starts with loss accumulation of insurance coverages from locations to policies to events. In this article we focus on the three aspects of this procedure: new loss distributions across different perils and insurance coverage types; coverage correlation, for which we use the same mathematical foundation as for the spatial correlation aspect of portfolio rollup as discussed in Part I; and support of new financial terms and conditions. After discussing these three aspects of portfolio rollup, we discuss the financial modelling of secondary perils in NGM, explaining how we have engineered all tropical cyclone, hurricane, severe thunderstorm, and earthquake models to support and produce secondary uncertainty distributions of damage ratios both by sub-peril and for all-perils combined. We then provide ground-up losses for each sub-peril, thus enabling you to model and price all possible combinations of contract coverage and terms.
Loss Distributions across Perils and Coverage Types in the NGM Framework
The entry point to our next generation financial module is the probabilistic uncertainty description for coverage loss, given in the form of the underlying loss distribution. One characteristic of such loss, estimated by catastrophe models, is the mean damage ratio (MDR) or, when multiplied by the property’s replacement value, the mean loss. This mean loss is a function of, for example, wind speed and exposure data linked by geographic location. In the right panel of Figure 1, the residential area shown was affected by the same wind speeds of Hurricane Laura and therefore with the same model-predicted mean loss, yet we see a range of corresponding damage to buildings. To account for this type of uncertainty, using structural engineering expertise, Verisk applies a vulnerability analysis to each individual property and, given the intensity of a simulated event, a probability distribution of damage is developed for the property at the policy coverage level, i.e., for buildings, other structures, contents, and time element. An example of a discrete probability density function (PDF) of the loss distribution is shown in Figure 2.
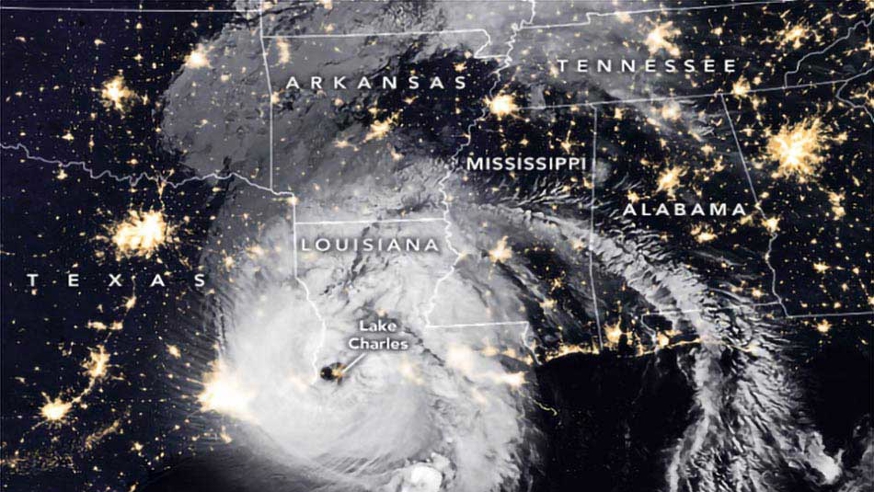
Figure 1 : Hurricane Laura, August 2020 (Source: NASA Earth Observatory).
Probability Density Function Features: Atoms and Main Part
The PDF in Figure 2 has two important practical features: The first is the discrete spikes called atoms at 0% damage ratio and 100% damage ratio, which represent the probability of no damage (shown in brown) and full damage (showed in green), respectively.
The spike at zero damage might be because:
- a particular exposure was not affected by a particular peril during a catastrophic event
- the damage was below the deductible
- the model-predicted footprint of the event did not match the actual footprint, causing mean damage to be slightly above zero for areas unaffected by the event
The spike at total damage might be, for example, attributed to:
- a house shifted off its foundation
- overall structure racking (when a building tilts out of plumb)
- irreparable structural damage (structure still partially intact)
- structural failure
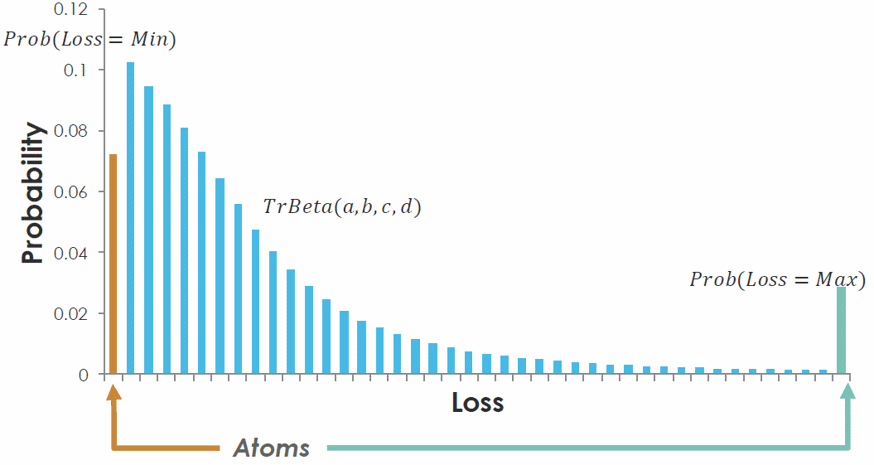
Figure 2. Discrete probability density function of loss. (Source: Verisk)
The second feature of a typical loss distribution is the main part (shown in blue), which models the bulk of the distribution for the damage ratios in between the two atoms. In the next generation financial module we use a discretized 4-parameter Transformed Beta family to describe the main part. Our choice was based on our analysis of insurance claims data. By normalizing the frequency of claim damage ratios, the uncertainty distribution for a given modeled MDR can be inferred. Accordingly, an empirical damage distribution is derived for each intensity and modeled MDR. These empirical relationships are then mirrored in a functional form of a 4-parameter transformed beta rather than, for example, less flexible 2-parameter distributions such as beta, gamma, or log-logistic. This allows for more accuracy in modelling the tail of the empirical loss distribution inferred from the insurance claims data.
The new distributions have smoothly transitioning shapes of the two spikes and the main part, as the MDR increases. The support of each distribution is a single connected interval—there are no large gaps with zero probability in the main part of the distribution. For example, consider a geometric spiral alignment, shown in the left panel of Figure 3, to plot a set of loss distributions for the hail peril for Coverage A. Moving around the spiral toward the center of this diagram is analogous to moving from the outskirts of a hailstorm toward the areas subject to the most intense hail downpours. Each distribution is colored by the value of its MDR using the heatmap in the right panel of Figure 3. White on the heatmap represents an MDR approaching 0, or no loss, while red represents MDR approaching 1, or maximum loss. As we move along the spiral, the distributions transition from the spike at zero damage, monotonically inflating the bell-shaped main part, which then smoothly shifts toward the spike at total damage in the middle of the figure. The increase in high levels of damage shown in the main part of the distributions stems from the fact that hail usually causes damage to exterior surfaces, including walls, roofs, doors, and windows, and impacts not only the appearance of these elements but also their function.
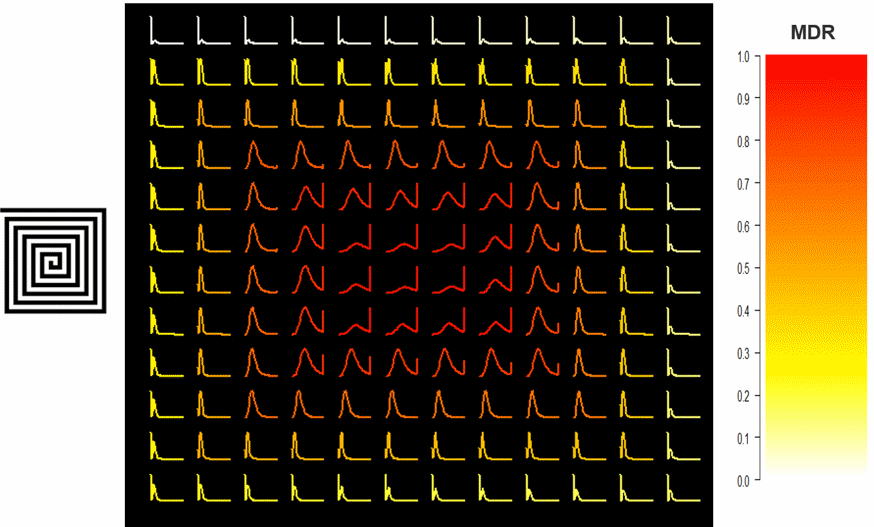
Coverage Correlation
To accurately represent uncertainty in the sum of coverage losses, the dependencies between losses for different coverage types need to be accounted for—for example, how strongly the damage to the contents of the building depends on the damage to the building itself. Currently, Verisk models capture these dependencies deterministically, in terms of predicted mean damage. In the next generation financial module, instead of simply adding MDRs (scaled by replacement value), we aggregate the four coverage loss distributions to a single location loss distribution in the following sequential actuarial order: A (building), C (contents), B (appurtenant structures), and D (time element). This is done using the mixture method for computing the distribution of the arbitrary sum > fS of dependent random variables, which we discussed in Part I of this series.
The sums of interest are referred to as the A+C, A+C+B, and A+C+B+D. Each time the loss engine adds one coverage to the sum of coverages, the mixture weight is used to obtain a new distribution characterizing this sum. The weight quantifies the strength of the comonotonic (maximally correlated) dependency between two random variables. The loss engine imports three weights w1, w2, w3 for A+C, A+C+B, and A+C+B+D, respectively. Then, the mixture method is applied sequentially using the following convolution-based scheme:
where ,
and
are the discrete PDFs describing (partial) sums of coverages and the superscripts “Ʇ” and “+” represent independent and comonotonic (maximally correlated) counterparts of coverage losses and their sums.
We can use statistical inference to estimate the weights of the independent and comonotonic functions by retrieving the pre-estimated loss distributions using the MDR for each coverage. Two loss distributions characterizing the damage to buildings and contents (coverages A and C) for a hurricane event are shown in the upper left and the upper middle panel of Figure 4.
The empirical PDF of their positively dependent sum A+C inferred from claims data is shown as the red curve. This positive dependence falls in between two bounds: independence (zero correlation) and comonotonicity (maximum correlation). So, if we assume that Coverage A and Coverage C losses are independent, the distribution of A+C is computed using numerical convolution (shown in the blue curve). On the other hand, if we assume that Coverage A and Coverage C losses are comonotonic (maximally correlated), the distribution of A+C is computed using numerical quantile addition (shown in the purple curve). The mixture method (shown in the green curve) computes the weighted combination of the independent and comonotonic case. The weight w should be chosen such that this combination matches the empirical distribution (red curve).
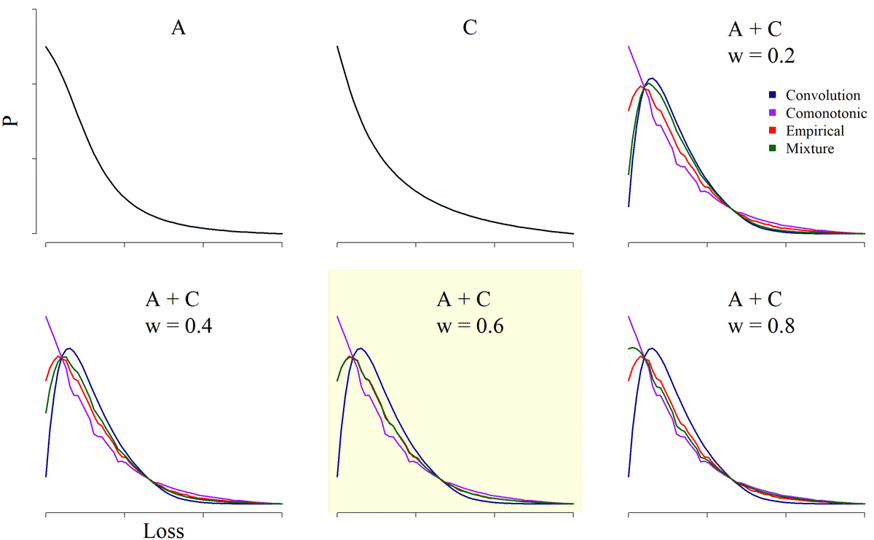
Figure 4. An example of weight optimization procedure. (Source: Verisk)
For w=0.2 the green mixture distributions approach the independent case, shown in blue. For w=0.4 the mixture has a shape more similar to the empirical distribution in red, but the match is not optimal. For w=0.6 the red curve is almost indistinguishable from the green one, showing an optimal match between the two distributions. If the weight is increased, bringing it closer to 0.8, the green curve starts to get closer to the comonotonic case in purple, resulting again in a sub-optimal match. The same optimization process is applied to obtain weights to accumulate coverages A+C+B and A+C+B+D.
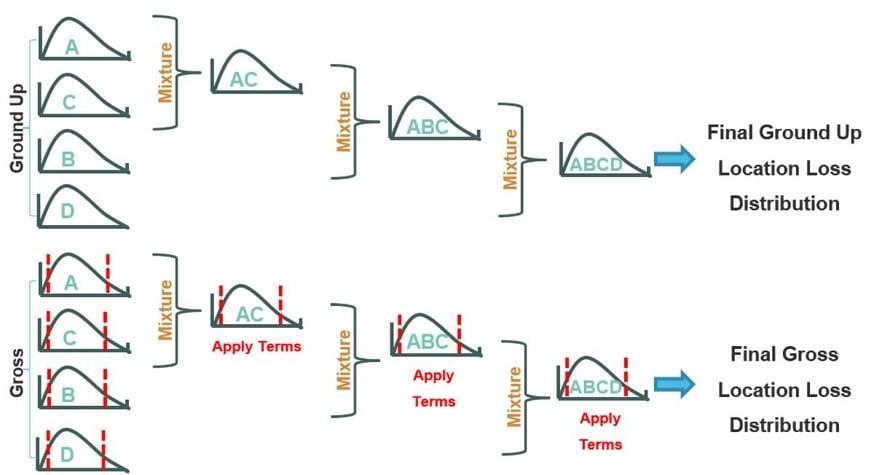
During portfolio rollup, the loss engine imports the three weights for A+C, A+C+B, and A+C+B+D from a hard-coded table. The values of the weights are a function of model MDR for Coverage A only. Then, the numerical scheme in the equation shared in the “Coverage Correlation” section is invoked and loss accumulation follows the workflow in Figure 5. So, we first integrate coverages A and C with the weight w1. For gross loss, if required by single risk insurance, financial terms are applied on this partial sum of losses. Then we cointegrate Coverage B with the weight w2, and again, if needed, financial terms are applied for gross loss estimation. Lastly, we add up Coverage D loss with the weight w3, again applying the financial terms on the partial aggregate of gross loss. In this way, we obtain final estimates of ground up and gross loss distributions for a location in a statistically sound way.
Financial Modelling for Secondary Perils
Modelling the insurance coverage of secondary perils, such as storm surge related to a hurricane or liquefaction related to an earthquake, has become increasingly important. To address this market development, we provide ground-up losses for each sub-peril, thus creating the ability to model and price all possible combinations of contract coverage and terms. In NGM, the model computes the corresponding losses and exceedance probability (EP) curves for each secondary peril. All of the new tropical cyclone, hurricane, severe thunderstorm, and earthquake models have been engineered to support and produce secondary uncertainty distributions of damage ratios by sub-peril and for all perils combined. These secondary uncertainty distributions from the peril models become exposed to the next generation financial module for all tiers and aspects of insurance loss modelling.
How Does It Work in the Verisk Hurricane Model for the United States?
For Verisk’s U.S. hurricane model, this new loss modelling framework allows you to propagate four loss distributions from the peril model to the financial module—three individual peril distributions for hurricane wind, storm surge, and precipitation-induced flood and the all peril distribution. You need to do parallel accounting of the policy all-peril loss and of the individual losses by sub-peril. In a typical policy case, the insurance coverage is placed on each of the secondary perils. The insurance policy terms and conditions—location deductibles and limits—are placed individually on each or on some of the sub-perils. The insurance losses by each location and by each sub-peril are computed accurately with modern actuarial methods. To reflect this in the all-peril loss distribution we use a statistical and fully probabilistic method to prorate the combined loss distribution with an aggregated insurance gross to ground-up ratio. Once this procedure is performed, coordination and synchronization are achieved for all our loss types, for both the all-peril and the single sub-peril type.
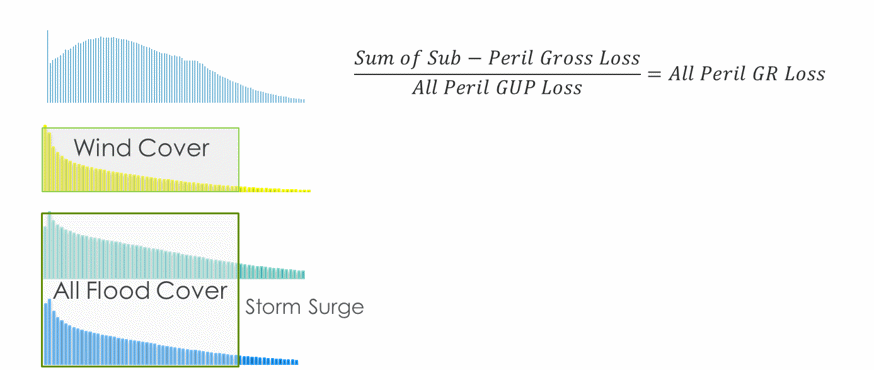
The insurance cover can also be placed on the all-peril ground-up loss. The combined, cumulative peril loss for the risk is insured. Your task as a modeler is to reflect and report the impact of this all-peril cover on the single sub-peril losses. In principle, the same prorating technique is used. An aggregated ratio from all-peril insurance gross and ground-up loss is created. Then, this ratio is used to probabilistically prorate each of the single sub-peril losses to reflect the impact of the top-level insurance cover. In principle, this is a pure back-allocation procedure to reflect the impact on component-peril loss types.
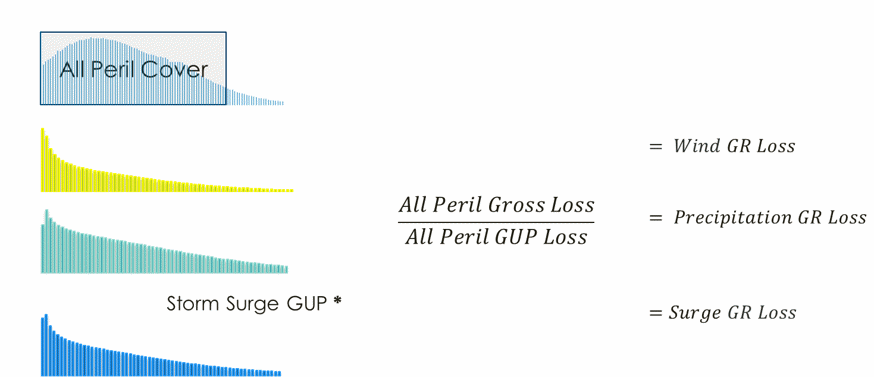
Verisk’s Next Generation Financial Module Supports New Terms and Conditions
The new insurance terms supported by Verisk’s next generation financial module falls into two general groups: annual aggregate terms and conditional terms. The modelling methodology for one of the most typical aggregate terms—the location and single risk annual aggregate deductible—is comprehensive. Two aspects of this methodology are important for our clients: first, it is fully probabilistic and can be validated with claims experience; second, it works for all catastrophe modelling cases—from single peril model analysis, such as winter storm or wildfire, to multi-peril models, such as tropical cyclone and earthquake, and beyond to multi-model analysis runs. This is possible because the day of year sequence of stochastic events within a modeled stochastic year is used for the chronological ordering of losses going into aggregate policies.
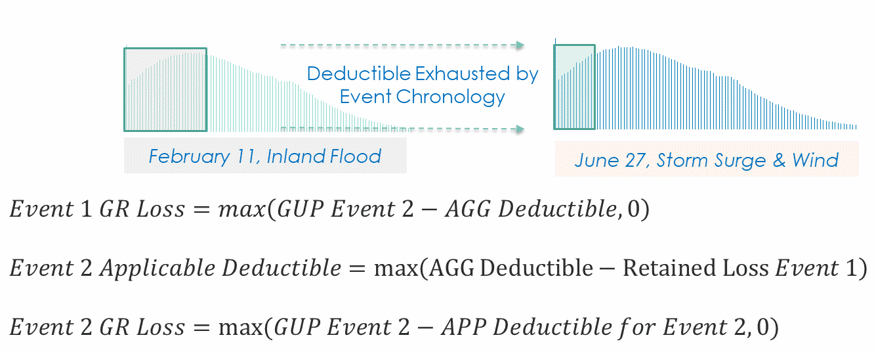
The deductible is applied on the first event of the year—irrespective of the peril in the general case of a multi-model run. The new generation of loss distributions are used and the loss accumulation from the insurance coverage loss to the site loss is done with coverage correlations. Next, probabilistic scenarios for the applicable aggregate deductible are created for the second peril event in the year, which is computed by exhausting the original aggregate deductible by the already covered retained loss from the first event. Lastly, these applicable deductible scenarios are deployed on the second event of the year. This procedure continues until all catastrophe events in the modeled year are covered.
The same approach is implemented for the second most typical aggregate term in the industry—the single risk, or location annual aggregate limit. The algorithm works from the single peril model case to the multi-peril and multi-model analysis run case.
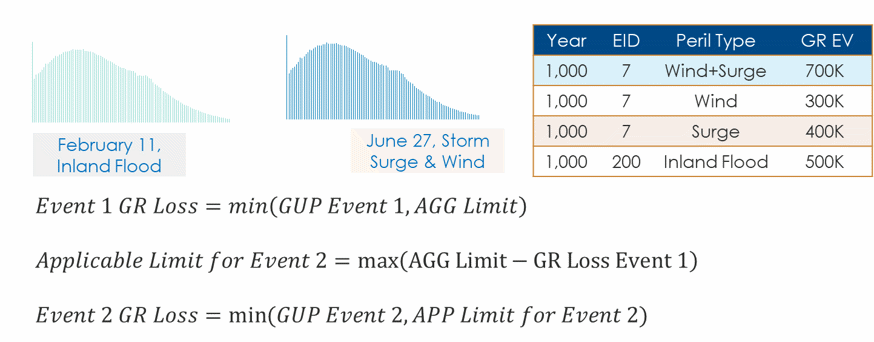
The aggregate limit is applied on the first event of the year. Then a probabilistic applicable limit scenario is created for the second consecutive event by exhausting the original value of the aggregate limit for the already covered insurance gross loss in the first event. Once these applicable limit scenarios are computed, they are used for the second event of the year; we continue to run the algorithm until all events in the year are covered. In the case of secondary-peril and multi-model analysis runs, all insurance gross loss is reported with individual records in the year-event loss table.
Next Generation Financial Modelling Implements a Third Tier for Aggregate and Conditional Terms
Modelling single-risk conditional and multi-tiered policies is particularly relevant for the small business lines segment. In our current financial module, we support two tiers of occurrence-based, location insurance terms, which are not conditional on other tiers of insurance but are applied sequentially. Most typically coverage terms are computed first, with site terms computed second. In our next generation financial module, a third tier of aggregate and conditional terms is implemented.
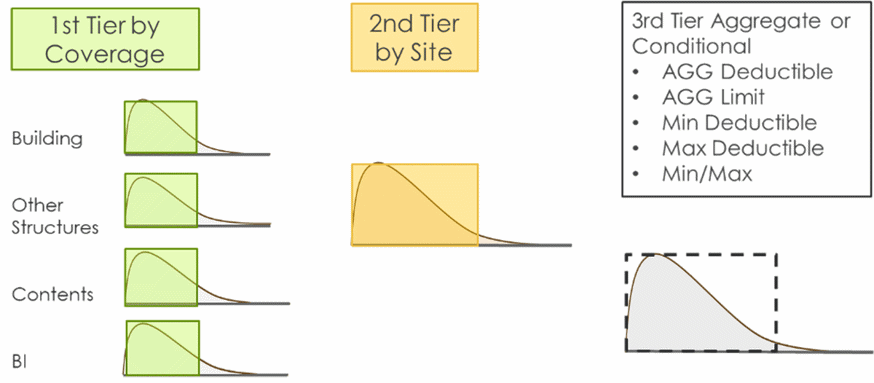
This is also the tier where you, the modeler, place your annual aggregate deductible and limit. These aggregate terms can be applied in combination with individual occurrence terms (captured in the first two tiers described above), but typically these types of aggregate terms are applied without additional occurrence terms also being in place. This enables you to accurately represent a typical annual aggregate policy.
A third tier of occurrence conditional minimum and maximum deductible types are also supported in the next generation financial module. These are conditional policies and terms because—based on the conditions of the policy and on the placing choices of the modeler—only one tier of deductibles will be applied, and thus the over-application and over-stacking of deductibles is avoided. A very typical representation of third-tier conditional occurrence deductibles is when there is a single risk policy with deductibles by coverage and limits by site or by coverage, and then a third tier conditional minimum, maximum, or min/max deductible type is also applied. This is a policy structure that we have developed to capture the insurance coverage we often see on single risk and small business lines.
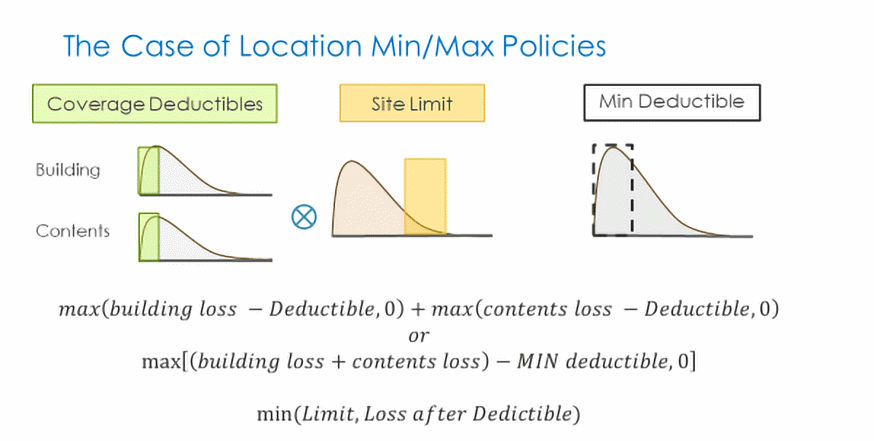
The intention of the modeler here is to have a selection procedure and apply one of the deductible tiers based on pre-specified conditions. For this purpose, two deductible scenarios are computed: one by applying the coverage deductibles; and the other by applying the min or max deductible on the ground-up loss. Based on the conditions of the policy, one of these scenarios is chosen and applied before coverage or site limits. With this new algorithm we can achieve actuarial accuracy and avoid over-application and over-stacking of deductibles.
Next Generation Financial Modelling Produces a Higher Fidelity View of Risk
In Part I we discussed our new loss accumulation methodologies with spatial correlations. With the implementation of these methodologies for all residential and small business risks within the catastrophe event footprint, we are able to propagate this enhanced single risk loss accuracy, and also geospatial dependencies, to the book of business level. Thus, by bringing together our next generation of work on the core principles of natural catastrophe modelling with statistical methodologies of loss accumulation, and the best industry practices for actuarial procedures and workflows, we are enhancing your ability to reflect a wider variety of contracts more accurately in the residential and small business markets and produce a higher fidelity view of risk. This higher fidelity application of policy terms results in a more realistic view of risk that is reflected from the single risk insurance coverage loss distribution, to the geographic accumulation, to the unit and full book of business perspectives.
Resources
Sharpe, James, (2008), Loss Models: From Data to Decisions. By Stuart A. Klugman, Harry H. Panjer & Gordon E. Willmot 3rd edition, John Wiley & Sons, 2008. 726pp. ISBN: 9780470187814, Annals of Actuarial Science, 3, issue 1-2, p. 327-333.
Venter, Gary. (1983). Transformed beta and gamma distributions and aggregate losses. Proc. Casualty Actuarial Soc. 70.
Wójcik, R.; Liu, C.W.; Guin, J. Direct and Hierarchical Models for Aggregating Spatially Dependent Catastrophe Risks. Risks 2019, 7, 54.